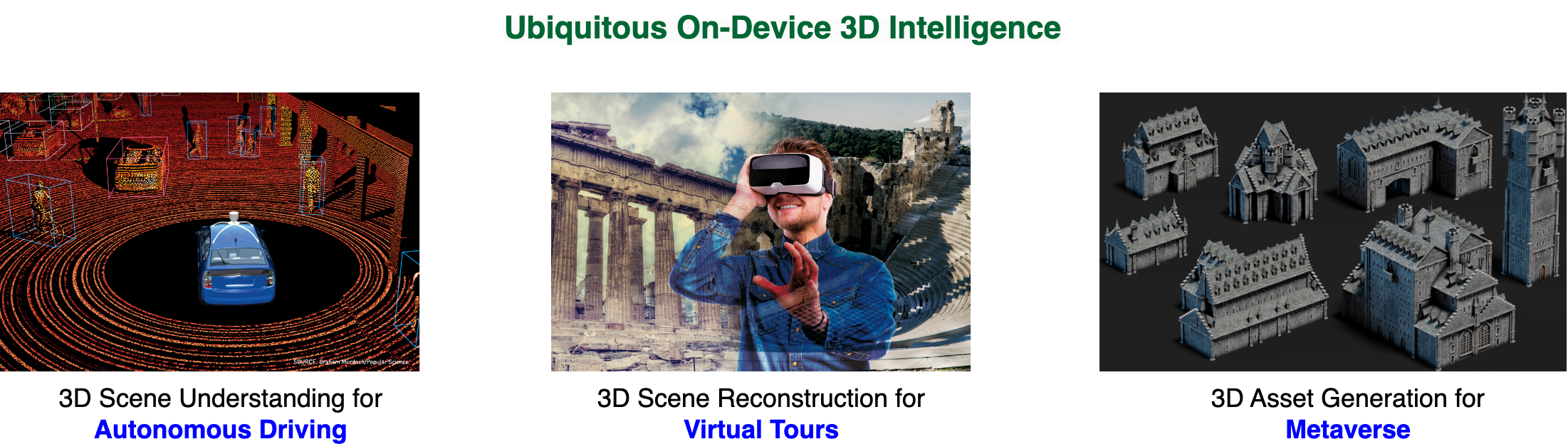
Image Sources: tinyurl.com/4uy268dh, tinyurl.com/ytmwknzu, and tinyurl.com/w69rmw5e
Cluster 1: Ubiquitous On-Device 3D Intelligence
Students: Chaojian Li, Sixu Li, Cheng-Jhih Shih, Zhifan Ye, and Yonggan Fu
3D intelligence is widely regarded as the next step in artificial intelligence, following text- and image-based applications. Many 3D intelligence applications today promise to deliver richer and more immersive experiences compared to those based solely on text/images. However, this greater promise of 3D intelligence comes with significantly increased computational complexity and memory storage requirements, which conflict with the limited resources of everyday edge devices. These challenges make it difficult to achieve ubiquitous 3D intelligence with the desired real-time response and high energy efficiency.
Our goal is to bridge the gap between the highly promising yet resource-intensive 3D intelligence applications and the resource-constrained everyday devices, thereby enabling ubiquitous 3D intelligence. Specifically, our research provides a holistic solution through co-design across architecture, algorithms, and systems, complemented by the development of corresponding community infrastructure.
You are welcome to join us as we tackle these groundbreaking challenges in 3D intelligence. Whether you're interested in architectural innovations, algorithmic optimizations, or system-level solutions, there are numerous opportunities to contribute to this emerging field. Together, we can work towards making sophisticated 3D intelligence capabilities accessible on everyday devices, transforming how we interact with and understand the world around us.
Corresponding Publications:
Cluster 2: Intelligent Eye Tracking
Students: Haoran You, Yang (Katie) Zhao, Cheng Wan, and Zhongzhi Yu
Eye tracking captures the position and movement of human eyes, enabling machines to interact with humans, especially in Augmented and Virtual Reality (AR/VR) environments. For example, foveated rendering, which depends on high-performance eye tracking, is a key technology for creating immersive experiences in AR/VR devices.
However, current eye tracking systems use bulky lens-based cameras, which pose challenges such as large form factors and high communication costs between the camera and the processor. These limitations make it difficult to achieve the high throughput needed (e.g., >240 FPS) for real-time human-machine interaction in resource-constrained AR/VR devices. The demand for integrating advanced AI algorithms, like deep neural networks, further complicates achieving high performance.
To overcome these hurdles, we are developing intelligent eye-tracking systems through a co-design of the camera, algorithms, and processor chips. Our approach aims to meet performance requirements without sacrificing accuracy. Join us as we pave the way for next-generation eye tracking solutions and drive future innovations in intelligent imaging systems.
Corresponding Publications: